About the Author
Data Quality – The Backbone of Successful Data Science. Even the best algorithms will often struggle to return useful results without clean, accurate, and consistent data.
Data that is incorrect is expensive — costing companies US$15 million every year on average, according to Actian.
With data being a major factor in the decision-making processes of organizations today, having high-quality data is indispensable for getting reliable insights, making better decisions, and fulfilling organizational goals.
Data Quality in Data Science: Practical Tips for Cleansing, Validation and Governance This blog explores how data quality is a crucial enabler of data science and provides practical advice on cleansing, validation and governance.
Plus, what new trends and technologies mean for data quality management and professionals looking to stay ahead in this constant state of innovation.
What Is Data Quality?
Data quality, in terms of a dataset, emphasizes a combination of accuracy, completeness and relevance among others. Data of good quality will guarantee good insight, and predictions to be relevant and reliable since it forms the underlying basis of all data science activities.
- Accuracy: Making sure that the data tends to be as error free as possible and corresponds to the real world.
- Completeness: Assesses if all required data components are available in the dataset.
- Consistency: Aims at ensuring uniformity across data sets in a bid to prevent contradictions.
- Reliability: Determines to what extent the data can be trusted in making decisions.
- Relevance: Refers to the data collected for a specific problem or evaluation if it is still useful for that particular case.
Why Data Quality Matters in Data Science?
While performing various functions in data science, one thing that has to be taken into serious consideration is data quality since it has a direct effect on the outcome of the algorithms and the predictive models constructed.
Without good quality data, the end results would be meaningless, and it would be wasting resources and losing faith in the data driven techniques.
- Impact on Machine Learning Models: Higher chances of unreliable and biased models being constructed due to bad quality of the dataset.
- Cost Implications: Errors resulting from poor data standards have and will cost organizations dearly. Gartner suggests that poor quality data costs organizations twenty million every year.
The Core Components of Data Quality
Data Cleansing
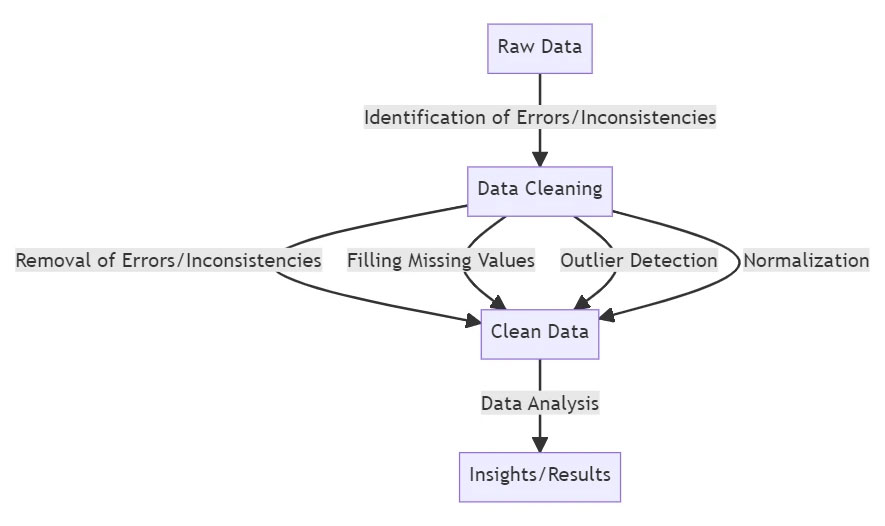
The data cleansing definition relies on the identification and assessment of errors and inconsistencies in the datasets. This is the case even with the removal of the irrelevant, duplicate or incomplete records.
Key Techniques in Data Cleansing:
Error Detection and Correction. Recognition of the data entry as an outlier or an incorrect data point that needs to be fixed.
Duplicate Removal. Removal of duplicate entries on the record to protect and keep the data set untainted.
Standardization. Ensuring that all values have the same meaning in these aspects (date, numerics and other units)
Benefits of Data Cleansing:
- Higher effectiveness of the models.
- Higher usability of the processes.
- Enhanced or improved understanding of the customer.
Data Validation
Data validation is an important activity that facilitates and ascertains that a dataset meets some specified particular set of standards and criteria before it gets applied for analysis.
Validation Methods:
Rule-Based Validation: Setting limitations and requirements to rules, for example a minimum and a maximum range of possible values or a compulsory column.
Cross-Validation: Using a dataset that is more reliable and trusted to compare and confirm other data.
Why It’s Important:
- Stopping unusable data from passing through different stages of analysis.
- Adhering to data governance as well as all the set rules and regulations.
Data Governance
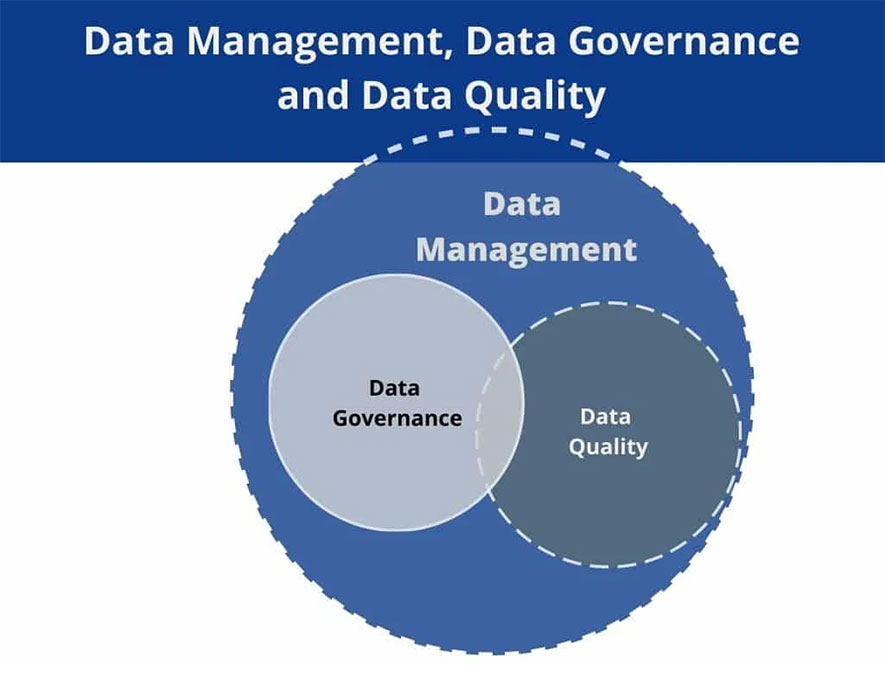
Data governance is the development of strategies and practices that work within an organization to ensure the protection and quality of data assets.
Governing data begins with:
Data Stewardship: Appointing people to look after data in terms of its accuracy and availability.
Policies and Standards: Establishing a framework for how data should be created, collected, held, and processed.
Technology Implementation: Availing software or hardware that embeds governance practices and reduces the workload.
Influences on Quality of Data:
- Facilitates accountability and transparency.
- Minimizes the chance of accidentally misusing data or subjecting it to a breach.
Best Practices for Maintaining Data Quality
1. Implement Data Cleansing Processes Regularly
The constant cleansing of data on a normal and routine basis should be the very first step in high quality data maintenance. Errors in a dataset such as duplicates, incompleteness, and aging of data tend to increase with time. With the help of a strong routine for data cleansing, these problems are fixed and the data cleansed to get rid of any inaccuracy or irrelevance.
Using software can assist with data cleansing automation, which would eliminate some of the manual work and increase efficiency. This means that businesses will not be held back by inconsistencies, allowing them to fully utilize their data in making decisions and analytics.
2. Establish Clear Data Governance Policies
The long-term quality of data should be governed by data governance. By creating rules that govern how data is collected, stored, and used, organizations could mitigate regulatory concerns around compliance.
Appointing data stewards or data governance teams can help in oversight of data quality indicators and the enforcement of these metrics across the departments. Proper governance promotes responsibility, the likelihood of making a mistake or abusing data is minimized, and trust in datasets is boosted.
3. Invest in Employee Training and Awareness
Data quality is not solely about tools and processes—it also depends on the people handling the data. Providing training to employees about the importance of accurate data entry and the impact of errors on business outcomes is crucial.
Employees who understand the value of quality data are more likely to follow best practices and adhere to guidelines. Regular workshops or e-learning modules can keep them updated on the latest data quality tools and techniques, fostering a culture that prioritizes accurate and reliable data.
Emerging Trends and Technologies in Data Quality
AI and Machine Learning in Data Quality Management
In today's world, it is difficult to imagine how organizations would ensure the highest level of data quality management without employing AI and machine learning.
Automated Anomaly Detection: This facilitates faster and accurate resolution of errors by correcting them on the go with the help of artificial intelligence algorithms which track activities carried out on the dataset.
Data Matching and Deduplication: Machine learning models improve the accuracy of record linkage by identifying duplicates and consolidating records with minimal manual intervention.
Predictive Data Quality: Using sophisticated AI models, one can identify the future possibility of a certain issue arising with the data quality and provide triggers for actions to be taken to avoid such instances.
These technologies reduce human error, save time, and ensure datasets are optimized for analysis.
Blockchain for Data Integrity
The essence of blockchain revolves around ensuring that the data will be correct and cannot be corrupted through a central system.
Immutable Records: With blockchain, once data has been added, it becomes impossible to change or manipulate that data thus conserving the chronological order of events for that database.
Enhanced Security: Because resources are pooled on a distributed ledger, the chances of hacking and making undesired changes to data are considerably lower.
Use Cases in Data Quality: Blockchain portrays its full potential for accuracy in industries such as healthcare and finance where accurate data entry is imperative.
Cloud-Based Data Quality Tools
Managing, maintaining, and scaling the quality of data within an organization can be easily handled with the use of cloud-only platforms.
Key Advantages:
- Capacity to Store High Amounts of Data.
- Minimized time lags when information is shared across teams and offices.
- Lower overall spending as compared to in-house installations.
Popular Tools: Tools like WinPure, Informatica, and Talend are very sought due to their easy to use dashboards and in depth data software.
Challenges and How to Overcome Them
Inconsistent Data Elements
Most systems have the same working functions but operate on different structures.
Example: One CRM might be in MM/DD/YYYY format, and another could be on DD-MM-YYYY.
Data Control Issues
Data privacy conforms to laws such as the GDPR and the CCPA with companies that have involved customers.
A data breach can result in horrendously large fines and tarnish a company’s reputation.
Large Capital Expenditure
Funding sources can be invested within tools and technologies which take a huge strain on a small business.
Strategies to Overcome Challenges
AI-Powered Tools
Use Multiple AI Based Tools to Easily Automate Data Standardization and Cleansing Without Consuming Time.
Gradual Progress Development
Begin with smaller data sets or focused campaigns and test their effectiveness and tweak strategies accordingly to scale them later.
Greater Confidentiality
Encrypt, limit permissions, and run compliance checks at regular intervals to secure sensitive data.
By addressing these challenges systematically, organizations can unlock the full potential of their data quality initiatives.
The Future of Record Linkage in Marketing
Integration of AI and Machine Learning
AI-driven algorithms have greatly impacted record linkage as they now increase the speed and accuracy of completing data-matching tasks. The use of AI allows for detecting complex relationships hidden within primary data and linking records from different sources.
This is all made possible by having real-time data as data processing becomes a reality increasing the quality of insights generated about customers.
With businesses now requiring speed and customization of their campaigns, AI and machine learning present a practical way for optimally linking records enhancing the chances of marketers achieving data-based campaigns.
Adoption of Customer Data Platforms (CDPs)
Advancements in technology and the growth of a number of channels customers are exposed to means that marketers need to adopt critical tools to manage and integrate customer data, and a CDP is such a tool.
When a CDP integrates data coming from various touch points, marketers can easily identify single customer data that can be fed into diversified marketing campaigns.
This gives marketers accurate and easy-to-target campaigns. All in all, CDPs improve the marketing tool integration thus making the CDPs data useful for marketing purposes.
The use of CDPs is integral in facilitating the alignment of data marketing because it enables the implementation of well-backed strategies.
Emphasis on Data Privacy and Compliance
Data Privacy has emerged as a crucial issue in the course of record linkage processes, these measures have been necessitated by regulations like the GDPR and the CCPA that turn ethical data handling into a requirement for all businesses that deal with people’s information.
There is a customer concern regarding how their data is fair and legal and to ensure fairness businesses go beyond complying requirements and turn to privacy standards.
Making sure that privacy is upheld when using the record-linking techniques increases the chances of businesses remaining in the law whilst creating a strong customer base.
In an era characterized by stiff competition, companies whose businesses are built on principles of privacy and data security are more likely to thrive in the market.
Conclusion
Everything revolves around data in this day and age. High-quality data translates to effective insights and more resources at your disposal.
In addition, it is good to clean, validate and maintain the data so that the insights can be actionable as well as dependable.
As such, it is important to remain abreast of new developments when it comes to trends in quality data management.
The critical fact is that one will want to pay attention to harnessing quality data in the first place to appreciate its impact in affecting projects, business performance, and success as a whole.