In the digital age, data is king, and data science has emerged as a pivotal field for extracting insights, making informed decisions, and driving innovation across industries. Data scientists, often referred to as data science professionals, play a crucial role in this landscape, utilizing advanced analytical techniques to uncover hidden patterns and trends within vast datasets. However, the efficiency and effectiveness of data science workflows heavily depend on the infrastructure supporting them. This is where cloud computing comes into play, revolutionizing the way data scientists operate and empowering them to harness the full potential of big data technologies. In this article, we will explore the profound relationship between data science and the cloud, highlighting the numerous benefits it offers to data science professionals.
The Intersection of Data Science and the Cloud
For those well-versed in the intricacies of the data science process, it's evident that a significant portion of data-related tasks is traditionally executed on a data scientist's local computer. Typically, this setup involves the installation of key programming languages such as R and Python, along with the integrated development environment (IDE) of choice. Additionally, essential components of the development environment, including relevant packages, are either installed through package managers like Anaconda or manually added to the system.
Once this development environment is configured, the data science journey commences, with data taking center stage. This iterative workflow typically encompasses the following steps:
- Building, Validating, and Testing Models: This phase involves creating and refining models, such as recommendation systems and predictive models.
- Data Wrangling and Transformation: Data must be cleaned, transformed, and prepared for analysis through tasks like parsing, munging, and cleaning.
- Data Mining and Analysis: This step focuses on extracting valuable insights from data, including summary statistics, exploratory data analysis (EDA), and more.
- Data Acquisition: Gathering the necessary data from various sources to fuel the analysis and modeling processes.
- Model Tuning and Optimization: Continuously improving and optimizing models or other deliverables to enhance their performance.
However, there are limitations to performing all data tasks on a local system, leading to several compelling reasons to explore alternative solutions:
- Processing Power:In many cases, the processing power (CPU) of the local development environment may be insufficient to execute tasks within a reasonable time frame or, in some cases, may not run at all.
- Deployment: To transition deliverables to a production environment or incorporate them into larger applications (e.g., web applications or SaaS platforms), a different approach is necessary.
- Data Size: Datasets can become too large to fit comfortably within the system memory (RAM) of a development machine, hindering analytics and model training.
- Efficiency: Utilizing a faster and more powerful machine with ample CPU and RAM resources is preferable to avoid overburdening the local development machine.
In such scenarios, various alternatives become available. Instead of relying solely on the local development machine, data scientists can offload computational work to either a cloud-based virtual machine (e.g. AWS EC2, AWS Elastic Beanstalk) or an on-premise machine. The advantage of employing virtual machines and auto-scaling clusters lies in their flexibility—they can be spun up and down as needed and can be customized to meet specific data storage and computational requirements.
Furthermore, alongside customized cloud-based or production-oriented data science solutions and tools, prominent vendors offer cloud- and service-based offerings that integrate seamlessly with popular tools like Jupyter Notebook. These offerings are often presented as machine learning, big data, and artificial intelligence APIs, and they encompass platforms like Databricks, Google Cloud Platform Datalab, AWS Artificial Intelligence platform, and numerous others.
These services facilitate data scientists' work by providing access to a robust and scalable cloud infrastructure tailored to their data science needs, allowing them to focus on extracting valuable insights from data without the constraints of local hardware limitations.
Top 7 Benefits of Using Cloud Computing in Data Science
The adoption of cloud computing in data science offers a multitude of benefits, transforming the way professionals work and improving the overall efficiency and effectiveness of their workflows. Let's discuss these advantages:
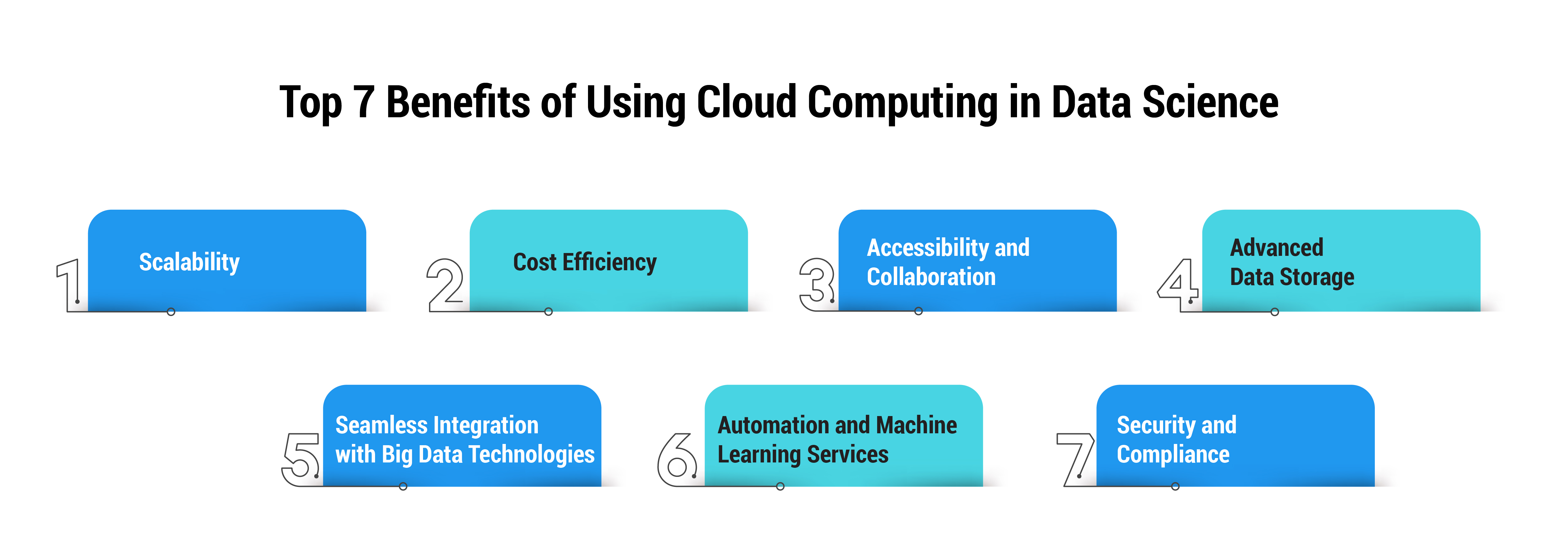
-
01. Scalability
One of the most significant advantages of the cloud is its inherent scalability. Data scientists often deal with vast datasets that require substantial computational power. With cloud computing, they can easily scale up or down based on their current needs. Whether it's processing large datasets, running complex algorithms, or conducting simulations, the cloud provides the flexibility to allocate resources accordingly. -
02. Cost Efficiency
Traditional on-premises infrastructure necessitates significant upfront investments and ongoing maintenance costs. In contrast, cloud computing operates on a pay-as-you-go model, where you only pay for the resources you consume. This cost-efficient approach eliminates the need for capital expenditures and allows data scientists to allocate their budgets more effectively. -
03. Accessibility and Collaboration
Cloud-based data science tools and platforms can be accessed from anywhere with an internet connection. This accessibility promotes collaboration among geographically dispersed teams of data scientists and other professionals. They can easily share data, collaborate on projects, and access the same resources, fostering innovation and knowledge sharing. -
04. Advanced Data Storage
Cloud providers offer a range of storage solutions optimized for various data types, including structured and unstructured data. Data scientists can leverage these storage options to efficiently manage and store their datasets, ensuring data integrity and easy retrieval when needed. -
05. Seamless Integration with Big Data Technologies
The world of data science often intersects with big data technologies like Hadoop, Spark, and Apache Kafka. Cloud platforms provide seamless integration with these technologies, simplifying the deployment and management of big data workflows. Data scientists can leverage cloud-based data lakes and data warehouses to store and process massive datasets efficiently. -
06. Automation and Machine Learning Services
Cloud providers offer a plethora of machine learning services and tools that streamline the development and deployment of machine learning models. These services come equipped with pre-built algorithms and automated model training capabilities, reducing the time and effort required to develop predictive models. -
07. Security and Compliance
Cloud providers invest heavily in security measures and compliance certifications. By migrating data science workflows to the cloud, organizations can benefit from robust security features, data encryption, and compliance with industry standards and regulations, ensuring the protection of sensitive data.
Conclusion
In conclusion, the integration of cloud computing into data science workflows has brought about a paradigm shift in the field. Data scientists, or data science professionals, are now empowered to tackle more complex challenges, process larger datasets, and collaborate seamlessly with their peers. The scalability, cost efficiency, and advanced features of cloud platforms have made them indispensable tools for data science practitioners.
As big data continues to grow in importance, the synergy between data science and the cloud will only become more pronounced. Organizations that embrace this synergy gain a competitive edge by unlocking deeper insights from their data, enhancing decision-making processes, and driving innovation. For data scientists, the cloud is not just a technological advancement but a catalyst for their professional growth and success in an increasingly data-driven world.
In this era of data-driven decision-making, data scientists must harness the power of cloud computing to stay ahead of the curve. By doing so, they position themselves as invaluable assets to their organizations and contribute significantly to the ever-evolving landscape of data science and big data technologies.