The age of big data is upon us. As organizations across industries accumulate vast troves of structured and unstructured data, "Data Science" has emerged as a crucial discipline to extract meaningful insights and drive innovation. Defined as the interdisciplinary field leveraging scientific processes and systems to derive knowledge from data, data science empowers decision-makers to base choices on hard evidence.
As we stand at the cusp of 2025, the future of data science is set for an exciting transformation, underpinned by rapid technological advancements. This article explores the key data science trends that will reshape data science in the coming years. Let's take a glimpse into the future!
Understanding Data Science
In simple terms, data science combines domain expertise, programming skills and knowledge of math and statistics to extract powerful and actionable insights from data. It helps answer critical business questions and enables data-driven decision making across industries.
From predicting customer behavior to powering fraud detection systems and personalizing healthcare, data science drives innovation and strategic thinking. The volume of data being generated globally is expected to grow at a stunning rate over the coming years. Tapping into this data asset efficiently will be key for continued innovation.
Growth Predictions for Data Science
As per industry reports, demand for data science skills is expected to grow by over 25% yearly over the next few years globally.
Key factors fueling demand are:
- Exponential data growth requiring advanced analytics at scale
- Increasing need to leverage data for strategic advantage
- Maturing AI and automation driving analytics innovation
- Growth in customer-facing applications powered by data science
This rapid growth underscores the critical role data science will play in digital transformation efforts across sectors.
Emerging Technologies Powering Data Science
Several supporting technologies closely tied to the evolution of data science are maturing rapidly. These innovations will shape what data professionals can achieve by 2025.
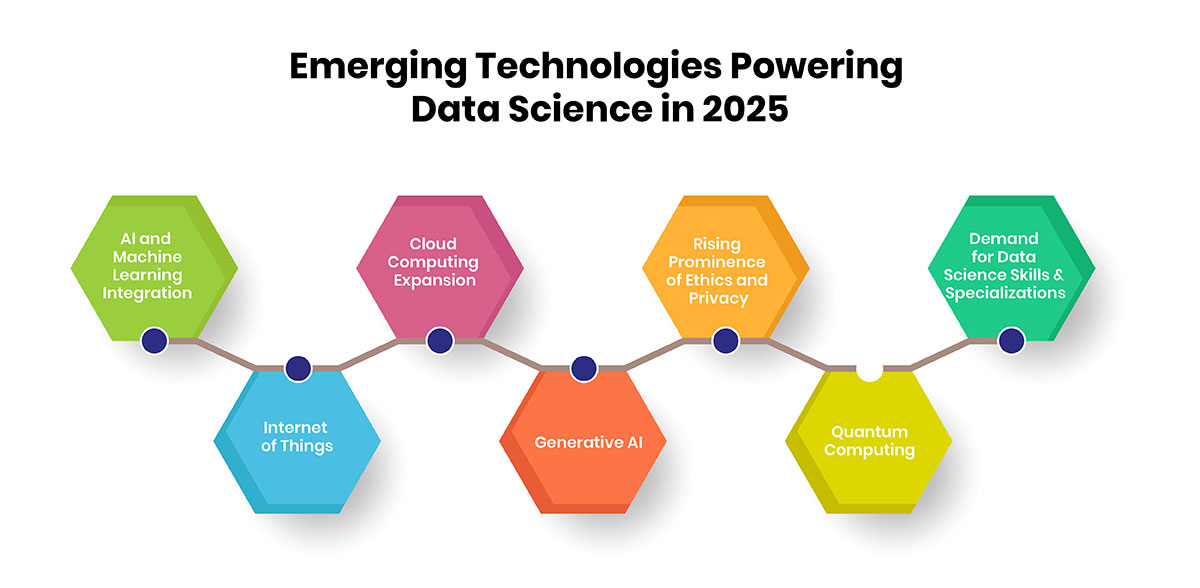
The Growing Integration of AI and Machine Learning
One of the foremost trends is the assimilation of AI and machine learning into data science workflows. As these technologies become more sophisticated, they will amplify the capabilities of data scientists and introduce process efficiencies.
Currently, data scientists spend considerable time on repetitive and manually intensive tasks such as data cleaning, preprocessing, feature engineering, and model building. AI and machine learning algorithms will automate such tedious work, allowing data experts to focus on higher-value responsibilities like solution design and business strategy.
Advancements in AutoML (automated machine learning) will also enable non-technical domain experts to benefit from machine learning. AutoML simplifies labor intensive processes like algorithm selection, hyperparameter tuning, and model optimization. It empowers businesses to leverage predictive capabilities without deep technical know-how.
As AI and machine learning get further ingrained in data science through innovations like AutoML, they will become indispensable parts of the field.
The Expanding Promise of Cloud Computing
The exponential increase in data requires scalable and flexible storage and computing solutions. This is where cloud computing delivers: offering vast storage capacity and computational horsepower to handle the deluge of big data. Cloud platforms like AWS, GCP, and Azure enable businesses to cost-effectively process gigantic datasets using data science and analytics tools.
As organizations grow wary of the overhead costs and complexities of on-premise infrastructure, cloud-based data science will gain more takers. The cloud's versatility also permits easy expansion or downsizing of storage and computing needs as demands evolve. By 2025, cloud-powered data science will become ubiquitous for cost and agility advantages.
While businesses can leverage pre-built AI tools on cloud platforms today, custom machine learning solution development on the cloud will surge too. Serverless computing presents new possibilities to run data science workloads in a dynamic, cost-optimal manner.
Rising Prominence of Ethics and Privacy
As data-driven AI decision-making permeates various walks of life, concerns around privacy, algorithmic bias, and responsible AI will take centrestage. Regulations like GDPR and CCPA already enforce transparency and accountability measures around using consumer data.
Data scientists will need to proactively assess and mitigate risks stemming from hidden biases in data or algorithms that can propagate discrimination. Techniques like differential privacy and federated learning preserve anonymity while deriving insights from sensitive user data.
Initiatives around explainable AI (XAI) will also gain traction to make AI systems more interpretable, ensuring fairness and transparency. Data practitioners will increasingly implement ethical frameworks comprising principles, policies, and best practices to steer developments in the right direction.
The Growing Demand for Data Science Skills and Specializations
As data matures from a business enabler to a mission-critical asset, organizations will aggressively invest in developing data science talent and teams. Industries are waking up to the multifaceted potential of analytics and AI across domains - from strategic planning to operations to customer experience.
Specialized data science roles will emerge to align solutions with specific business functions. Data scientists may operate as customer analytics experts in marketing teams or as predictive maintenance leads in manufacturing units. Instead of siloed functions, they will collaborate extensively with business stakeholders and technologists to shape products and processes.
Data science managerial and leadership roles will also rise to coordinate complex organizational initiatives. As tools and technologies evolve rapidly, developing a flexible, future-ready data science workforce will be crucial for organizations to stay competitive.
Internet of Things
The Internet of Things (IoT) is one of the most significant emerging trends that will shape the future of data science in 2025 and beyond. As more devices get connected to the internet, the amount of data generated through IoT sensors and devices is growing exponentially. By 2025, analysts predict there will be over 27.1 billion IoT devices worldwide, up from around 13 billion in 2021.
This deluge of real-time telemetry data from billions of sensors and devices will drive unprecedented demand for data analytics, creating major new career opportunities for data scientists. The global IoT market is forecasted to grow to $1.6 trillion by 2025 as industries invest heavily in connecting equipment, vehicles, infrastructure and more to derive value from IoT data.
Data science will be critical to realizing the full potential of IoT across sectors like manufacturing, transportation, healthcare, energy and smart cities. IoT data analytics will help uncover insights to optimize operations, prevent equipment failures, improve logistics, conserve resources and even save lives. Data scientists will develop machine learning algorithms to detect anomalies in IoT data that provide an early warning for critical events. Predictive maintenance analytics will help industries significantly cut downtime costs by fixing or replacing equipment right before failure.
Generative AI
Generative artificial intelligence (AI) is one of the most promising areas of innovation in data science. This technology uses neural networks to generate new, realistic data like text, images, video and audio. Over the next few years, generative AI is likely to see rapid advancement and increasing real-world application.
By 2025 and beyond, generative AI has the potential to transform many industries by automating creative tasks. In marketing for example, generative AI could create personalized ad campaigns, targeted promotional emails, and customized product recommendations for each customer. Retailers may even use AI to generate photorealistic images of products tailored to a shopper's taste. This would greatly improve customer experience and conversion rates.
Generative AI also has intriguing possibilities in sectors like healthcare and pharmaceuticals. AI models could analyze disease and treatment data to generate new drug candidates. They could also simulate how medications may interact within the body based on a patient's genetics and medical history. This could significantly accelerate the drug discovery process.
There are also defensive applications of generative AI in fields like cybersecurity. Models can be trained to generate realistic hacked content, system intrusions, or other threats. By exposing defenses to a wide diversity of attacks in simulation, security tools can become more resilient to actual hacking attempts.
Quantum Computing
Quantum computing is an emerging technology that harnesses the power of quantum mechanics to perform computations in fundamentally new ways. In the coming years, quantum computing is predicted to have a major influence on the future of data science and machine learning.
Rather than storing information using binary bits like classical computers, quantum computers use quantum bits or qubits which can exist in a superposition of states. This allows quantum computers to process exponentially more information simultaneously compared to even the most powerful supercomputers today.
By leveraging principles like entanglement and interference, quantum machine learning algorithms will be able to analyze intricate datasets and identify complex patterns on a massive scale. Tasks like optimization, personalization, predictions, and recommendations could be revolutionized using quantum-enhanced machine learning.
For instance, quantum computing may enable optimization across countless permutations of variables to find the best possible solutions for complex optimization problems in logistics, finances, manufacturing, and more. It could also enable ultra-precise recommendations by identifying patterns in consumer behavior from expansive datasets.
Personalized medicine is another key application - by scanning molecular and genetic data, quantum machine learning models may reveal connections missed by classical techniques. This can pave the way for specialized diagnosis and treatments tailored to individuals.
While universal quantum computing is still years away, leading technology companies and research institutions are making rapid strides. As quantum hardware and software mature over the next decade, we will witness this exotic field permeate into mainstream data science. The future of leveraging data will be defined by the symbiosis between quantum and classical techniques.
Exciting Times Ahead!
The field of data science is primed for major advancements through the assimilation of new technologies like AI, cloud computing, and advanced analytics. As organizations place data and insights at the core of their evolution, specialized data science skills will be highly coveted. However, we need concerted efforts to guide developments in an ethical and responsible manner.
As we stand at the edge of a hyperconnected world driven by intelligence derived from data, the possibilities seem endless. Indeed, the future of data science looks rather exciting!